The Cognitive Burden of Technology
Imagine it’s 7:00 a.m. Your phone, lying quietly on your bedside table, suddenly lights up, and your alarm rings. You sit up, turn off the alarm, and pick up your phone. Before you fully awaken or tend to basic needs, you’re bombarded by morning notifications: an urgent email from your manager, a panic-inducing alert from your crypto app warning about the market, a friendly nudge from your fitness tracker to hydrate and take 10,000 steps (because, of course, it knows you’re not moving). And don’t forget your social media updates. You swipe them away, but more keep coming like a persistent, invisible crowd trying to get your attention.
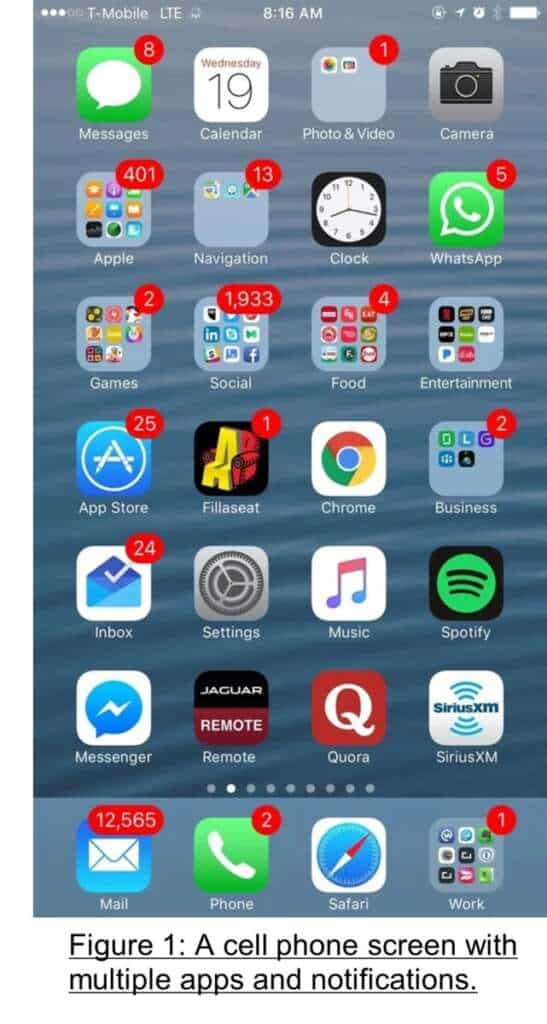
By noon, you’ve made dozens of tiny decisions: What’s today’s weather so I can choose my clothes? Which of my manager’s emails to prioritize? Should I skip breakfast or squeeze in a quick workout? As the day trudges on, your cognitive bandwidth declines, and by evening choosing what to watch on Netflix feels like a monumental endeavor. It’s no longer about “Should I have chicken or fish for dinner?” but a flood of choices that make deciding trivial matters overwhelming.
This isn’t just a personal anecdote—it’s a shared experience in the modern digital age. Technology, designed to simplify our lives, has inadvertently created a new kind of stress: information overload. With constant access to devices and endless content streams, we drown in choices that demand our attention. As remarkable as it is, the human brain wasn’t biologically built to process a relentless influx of data. Instead of empowering us, technology often leaves people feeling fragmented, fatigued, and overwhelmed.
Cognitive strain is bad for individual well-being and also for the very systems we design. Decision fatigue and analysis paralysis are the cumulative exhaustion from making too many choices, which undermines the effectiveness of technology meant to help us. Cognitive strain erodes trust, reduces engagement, and diminishes the user experience. In the pursuit of innovation, designers have overlooked a critical question: How can technology alleviate, rather than amplify, the mental burden it creates?
In my perspective, the fields of behavior change design and anticipatory design can be an asset in reducing cognitive strain, which occurs when our brain is burdened by multiple mental calculations or influenced by negative emotional states.
Behavior change design focuses on empowering users to form better habits and make healthier choices. Anticipatory design, alternatively, aims to anticipate decisions entirely by predicting users’ needs and acting on their behalf. Together, these approaches may revolutionize how users interact with AI technology—transforming it from a source of stress into a tool that acts like a helpful partner rather than a demanding child.
For designers, the challenge is clear: How can we design AI-driven systems that not only understand human behavior but actively reduce cognitive strain? How can we balance automation with agency to offer users relief from decision fatigue without stripping users of control? These questions are at the heart of the UX discipline today as we grapple with the psychological consequences of the digital age.
The Promise and Risk of Anticipatory Design
Anticipatory design has roots in both philosophy and psychology; it emerged as a solution to the growing problem of information overload. In the nineteenth century, philosopher Søren Kierkegaard described angst as the existential anxiety that comes from an overwhelming number of choices. In 2004, psychologist Barry Schwartz echoed Kierkegaard’s sentiment in his book, The Paradox of Choice (2004), arguing that too many options diminish decision-making capability and satisfaction. This insight laid the foundation for anticipatory design, which seeks to alleviate the burden of choice by predicting and simplifying decisions before users are even aware of their needs.
Building on this notion, Aaron Shapiro, CEO of Huge™, brought anticipatory design into the digital age, outlining its potential in his Fast Company article (2015); Shapiro defined anticipatory design as “responding to needs one step ahead.” By minimizing cognitive load, anticipatory design can eliminate unnecessary decisions, creating smoother, more intuitive experiences. This approach, aligned with AI, promises to streamline user experience by eliminating unnecessary choices, ultimately enhancing user satisfaction.
In the quest to create seamless experiences, anticipatory design gained attention in many businesses due to its promise to simplify daily decision-making processes either on a personal or professional level. Despite its significant promise, I wrote in Smashing Magazine about how “anticipatory design often fails to meet expectations when implemented in real-world contexts” (2024). This disconnect arises not from the limitations of the technology itself but from a fundamental misunderstanding of human behavior and the core principles of effective design. While AI systems can predict user needs, they often fail to account for the complexities of human decision-making and preferences, leading to mismatched expectations and user frustration. A deeper understanding of user psychology, combined with transparency, explainability, and user control, is necessary for anticipatory design to truly enhance the user experience, rather than hinder it.
As an advocate for human-centered AI, I find myself exploring why businesses often fail to meet users’ expectations. The exploration requires examining the psychological aspects of user experience and also the risks associated with over-automation. Although anticipatory systems are designed to predict user needs, they can inadvertently undermine user autonomy, leading to diminished trust and disengagement. To make anticipatory design work, designers must do more than predict user needs. We must understand human psychology. Transparency and user control are key to making these systems successful. Without them, anticipatory design risks becoming a double-edged sword—offering convenience on the surface but ultimately eroding trust and user autonomy. In essence, anticipatory design must prioritize human behavior and context to truly enhance the user experience and drive business value.
The High Expectations of Anticipatory Design
Anticipatory design operates on the principle that by analyzing user data—such as past behaviors, preferences, and contextual factors—systems can predict and fulfill needs before they arise. Ideally, this approach minimizes friction and boosts user satisfaction by delivering seamless, proactive experiences. Businesses are naturally drawn to this model as it promises smoother interactions, enhanced customer loyalty, and valuable insights through data collection. However, in practice, the implementation of anticipatory design often reveals unintended consequences that counteract its benefits. The over-automation of user experiences can inadvertently reduce a sense of control, leading to frustration, misaligned expectations, and a loss of trust. Anticipatory design has the potential to revolutionize user experience in the following ways:
- Reducing information overload: Presenting relevant information and streamlining options.
- Mitigating decision fatigue: Minimizing the number of choices users need to make.
- Promoting well-informed decisions: Framing information to avoid exploitation of cognitive biases.
- Creating efficiency and saving time: Automating repetitive tasks and streamlining processes, freeing up users’ time to focus on more strategic and creative tasks.
Although predicting user needs sounds appealing, it assumes a level of predictability in human behavior that doesn’t always exist, ultimately undermining the personalization that businesses seek.
Balancing Autonomy and Automation
One core principle of anticipatory design is to reduce the number of choices presented to users; the aim is to alleviate decision fatigue. However, this well-intentioned approach can backfire. Decision fatigue is a real concern, yet users often value the freedom to explore options and make informed choices. Here’s the conundrum: Simplifying choices too much can make them feel restricted or even coerced, leading to frustration and a sense of lost control. This is particularly true in contexts in which users expect to be actively involved in decision-making processes. For instance, a fitness app that suggests workouts based on past behavior might seem convenient by automating the same decisions, but users may feel they’ve lost the ability to choose the activity based on what they like to do at that moment. Over-simplification can undermine user satisfaction and engagement.
To succeed, anticipatory systems must balance automation with user agency. So, I built upon the Kaushik et al. (2020) framework of the ten levels of automation, which provides a valuable foundation for understanding autonomy in AI systems. However, their approach lacks a conceptual exploration of key factors such as the dynamics of human-AI collaboration and the varying levels of trust required at each stage. Kore (2022) addressed the trust dimension in his book Designing Human-Centric AI Experiences, but none of these authors have thoroughly examined the levels of automation in the context of human-AI collaboration. To address this gap, the table below maps system autonomy alongside the roles, responsibilities, and trust required for both humans and AI across these levels.
Table 1: System Autonomy versus Human Input
Level | Description | Human Role | AI Role | Shared Responsib-ility | Level of Trust Needed |
1 | No AI: Humans make all decisions. | Full decision-making and execution. | None | None | None (No AI involvement). |
2 | AI offers a complete set of alternatives. | Evaluate all options and make the decision. | Generate and present all possible alternatives. | Information gathering and presentation. | Low: Human retains full control. |
3 | AI narrows down a few alternatives. | Select the best option from AI’s suggestions. | Filter and refine the list of alternatives. | Decision refinement and risk evaluation. | Low-moderate: Trust in AI’s filtering ability. |
4 | AI suggests one decision. | Evaluate the suggestion and decide to act. | Propose the most optimal single decision. | Validation of AI suggestions. | Moderate: Trust in AI’s ability to identify the best option. |
5 | AI executes with human approval. | Approve or reject the AI’s proposed action. | Execute the action once approval is given. | Agreement on execution criteria. | Moderate-high: Trust in AI’s execution capabilities. |
6 | AI allows veto before automatic decision. | Monitor AI decisions and veto if necessary. | Execute decisions automatically after delay. | Define veto criteria and monitoring feedback. | High: Trust in AI’s ability to act without human interference. |
7 | AI executes automatic-ally and informs human. | Set parameters for AI decisions; monitor outcomes. | Execute actions autonomously and notify results. | Ensure decision criteria are met. | High: Trust in both execution and reporting. |
8 | AI informs human only if asked. | Rarely involved; intervene only on request. | Autonomously act and store justification data. | Define edge cases for human intervention. | Very high: Trust that AI can act without oversight. |
9 | AI informs human only if system decides. | Passive observer unless system signals issues. | Fully autonomous, decides when to inform human. | Exception handling for critical decisions. | Extremely high: Trust in AI’s judgment on when to involve humans. |
10 | Full autonomy: AI ignores the human. | No involvement: addresses failures if needed. | Fully autonomous, acts without human input. | Pre-set system design and regulatory oversight. | Absolute: Trust AI to function independently and reliably. |
Level 1–2 (no AI) involves systems that provide no assistance; humans are fully responsible for all decisions and actions. No trust in AI is required. As automation increases in Levels 3-6 (augmentation), AI begins to provide suggestions or narrows down decisions for human approval. These stages emphasize human-in-the-loop interactions, requiring moderate levels of explanation to build trust and ensure accountability.
In the highest levels of automation, Levels 7–10, AI takes on full autonomy by making critical decisions and informing humans only when necessary. These systems demand comprehensive explanations to ensure transparency, safeguard user trust, and validate the AI’s capabilities. The goal is to calibrate automation through well-timed, context-sensitive explanations, ensuring that AI is viewed as a reliable collaborator rather than an autonomous decision-maker. User control calibration not only enhances user experience but also supports safety by emphasizing human oversight. This aligns with the principles outlined in the ISO/IEC 42001:2023 checklist, which provides a framework for managing trustworthy AI by integrating risk management, accountability, and transparency into AI lifecycle processes (ISO 2023).
This is especially relevant as the new EU AI Act regulations, which focus on transparency and human control, seek to build trust in high-risk AI systems. The regulations reinforce the need for clear explanations and robust oversight to prevent misuse or disuse. Alternately, the Organisation for Economic Co-operation and Development (OECD) has created five value-based AI principles to promote the use of innovative but also trustworthy AI solutions that can respect human rights and democratic values.
The Science of Behavior Change
Today, as designers, we have to operate at the crossroads of psychology, technology, and UX. At its core, we need to learn how to leverage behavioral science to help users adopt healthier or more positive habits, make smarter decisions, and achieve long-term goals. Notably, in frameworks like Fogg’s Behavior Model (B=MAP) (2009), a psychological framework is designed to explain and predict how human behaviors occur. B=MAP has been particularly influential in persuasive design, behavior change, and human-computer interaction. According to Fogg (2009), Behavior (B) is the result of three interacting elements.
B=MAP
- M: Motivation—The degree to which a person wants to perform the behavior.
- A: Ability—The ease or difficulty of performing the behavior.
- P: Prompt—The trigger or cue that initiates the behavior.
“Behavior happens when Motivation, Ability, and a Prompt come together at the same time. When a behavior does not occur, at least one of those three elements is missing” (Fogg 2009).
This model emphasizes that designing for behavior change requires careful consideration of all three elements. Fogg argues that, for a behavior to occur, all three elements must be present, simultaneously and at sufficient levels. If one element is missing or inadequate, the behavior is unlikely to happen.
- High motivation and ability, but no prompt: No behavior occurs. The user does not know when or how to act.
- Strong prompt and high motivation, but low ability: Behavior is unlikely to happen due to difficulty.
- Clear prompt and high ability, but low motivation: Behavior may not occur because the person does not care enough. The user lacks the drive to engage in the behavior.
As Fogg (2009) states, behavior is not merely a result of intrinsic willpower but a product of well-designed systems that align motivation, simplify ability, and deliver timely prompts. Therefore, to effectively design for behavior change and anticipatory design, in the age of AI, I think we should borrow from behavioral science two additional frameworks: the Transtheoretical Model (TTM) (Prochaska and DiClemente 1983), also known as Stages of Change, and Nudge Theory (Thaler and Sunstein 2008).
TTM represents a person’s readiness to change, and Nudge Theory is its innovative approach to influence behavior. It advocates subtle interventions guiding individuals toward better choices without imposing restrictions. We should examine these complementary frameworks because AI amplifies behavior change processes by offering features like real-time feedback, personalization, and automated nudges.
Therefore, complementing B=MAP with TTM provides a stage-based approach to understanding behavior change. It recognizes behavior change as a process that unfolds over time, involving progress through a series of stages. TTM conceptualizes behavior change as a process involving progression through stages:
- Precontemplation: Not ready
- Contemplation: Getting ready
- Preparation: Ready
- Action: Making changes
- Maintenance: Keeping up changes
Take, for example, a user in the pre-contemplation stage. They might benefit from gentle awareness-building prompts, whereas a user in the action stage might need frequent reinforcement to sustain their behavior.
In my PhD dissertation, “Designing the Future: Exploring the Dynamics of Anticipatory Design in AI-Driven User Experiences” (Cerejo 2025), I provide a notable example of an AI anticipatory experience that failed to address the full spectrum of user stages.
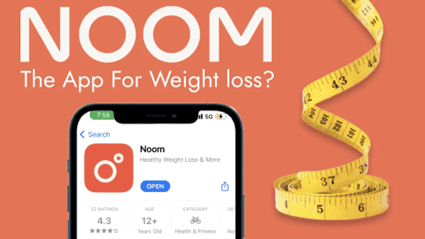
Figure 2. Representation of an app service for weight loss (App Store®)
The Noom® app is a digital health app designed to support weight management and healthy living. Although Noom’s AI-driven platform excelled at targeting users already in the action stage—providing detailed, structured coaching, and tracking tools—it struggled to engage users in earlier stages, such as pre-contemplation and contemplation. These users, who might not have yet recognized the need for change or who were still considering their options, were often met with rigid, overly prescriptive recommendations that lacked the subtlety needed to build initial motivation or foster commitment.
The app’s limited effectiveness extended to the maintenance stage as well. Its approach often lacked the adaptability required to support users in sustaining long-term behavioral changes. Instead of evolving alongside users’ shifting goals and routines, it relied on generic coaching strategies that failed to account for individual progress or setbacks over time. As a result, many users reported disengagement after completing their initial goals with limited support to prevent relapse or reinforce positive habits in the long term.
This case underscores the critical importance of designing behavior change systems that address users across all stages of the TTM framework. Interventions must be stage-appropriate. Designing for behavior change requires aligning nudges and interventions to users’ stages, ensuring relevance and adaptability. By failing to do so, even the most sophisticated AI anticipatory systems risk losing their effectiveness and long-term user engagement.
Complementing B=MAP with TTM enables designers to map motivational and ability-related interventions onto a temporal framework that captures the fluid nature of behavior change. However, the complexity of AI-driven solutions demands more than just these frameworks. To fully realize the potential of TTM, AI solutions must align with the psychological needs of users across all stages while leveraging advanced capabilities like real-time feedback, predictive analytics, and personalization.
That is why I also recommend integrating TTM with other behavioral science frameworks such as nudge theory to advocate for subtle, timely interventions that guide individuals toward better choices without imposing restrictions. Thaler and Sunstein’s (2008) principles for effective nudges include:
- aligning incentives with desired behaviors,
- providing immediate feedback to reinforce actions,
- simplifying choices for complex decisions, and
- making goals and progress visible through feedback loops.
These elements turn nudges into powerful tools that support behavior change and sustain motivation. Personalized nudges, rooted in data and attuned to the user’s stage of change, increase the likelihood of sustained engagement and effective learning. By integrating B=MAP, TTM, and Nudge Theory behavior models, designers can address gaps such as early-stage engagement, long-term adaptability, and transparency. Designers can formulate a set of guiding principles for effective behavior change design:
- Dynamic adaptability: Design systems that evolve with user behaviors, incorporating feedback loops and recalibration.
- Stage-specific engagement: Address pre-contemplation through exploratory prompts and maintenance through personalized, evolving strategies.
- Transparency-driven trust: Foster user confidence by making predictions explainable and actionable.
In anticipatory design, trust is critical as users must believe the system has their best interests at heart.
Core Principles of Behavior Change Design for AI Solutions
By leveraging these frameworks and principles, designers can create AI-driven solutions that are not only effective but also empathetic to the complexities of human behavior. Reflecting on the previous behavior frameworks, we may infer that there are three core principles of behavior change that we can use in design—user agency, personalization, and timely interventions.
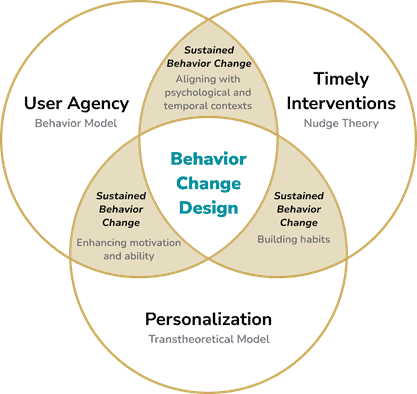
Figure 3 – My interpretation of how the proposed frameworks can interact to effectively design anticipatory experiences. The illustration shows how these elements combine to foster effective, user-centered behavior change systems.
If we combine the previous frameworks, we now have an effective way to design successful anticipatory experiences that sustain behavior change:
- User agency emphasizes control and autonomy to foster trust. “Behavior happens when motivation, ability, and prompt(s) converge” (Fogg 2009).
- Personalization adapts to users’ stages of change to align with their needs. “Behavior change unfolds in phases” (Prochaska and DiClemente 1983).
- Timely interventions guide users effectively to make decisions at the right moment. “Timely reminders subtly guide users” (Thaler and Sunstein 2008).
The first principle, user agency, suggests that users must feel they have control over their actions. If users think that an AI system is opaque (that is, coercive or manipulative), they are less likely to engage with it effectively. Empowering users to make decisions—even when nudged in a particular direction—helps maintain their autonomy while guiding them toward better choices. Fostering trust by allowing users agency in the AI system is crucial to building long-term engagement.
The second principle, personalization, emphasizes that behavior is not static; individuals progress through different stages when adopting new behaviors. Personalized interventions tailored to a user’s stage of change are more impactful than generic, one-size-fits-all interventions. This principle is deeply embedded in behavioral psychology, in which messaging and interventions tailored to individual needs are shown to be more effective in sustaining behavior change. For instance, a learning app might adapt its difficulty level according to the user’s proficiency, personalizing the experience for maximum engagement.
Finally, when designing anticipatory experiences, it is essential to consider the principle of timely interventions drawing on the concept of nudging. Nudging is especially important for anticipatory design because timely, context-aware interventions are at the heart of what makes anticipatory systems effective and user-centric. Anticipatory design relies on predicting user needs and acting preemptively, but without nudges that are well-timed and subtle, these predictions can feel intrusive or irrelevant.
The theory advocates for interventions that are delivered at the right moment—when the user is most receptive—to encourage positive behavior without coercion, which significantly increases the likelihood of desired behaviors. In anticipatory design, nudges are used to give timely, context-aware interventions, which make anticipatory systems user-centric and effective.
User Agency in Anticipatory Design
Over-automation can lead to frustration or distrust, so it’s essential to offer flexibility and transparency. For example, a learning app should explain why certain reminders or lessons are being pushed: “We noticed you tend to have more time to study after lunch, so we’ve suggested a review session around 1 p.m.” This helps users understand the logic behind the recommendation, which is transparent and explainable.
Similarly, a smart home system that anticipates the user’s ideal room temperature can offer a recommendation but must provide a clear option to override or adjust settings based on the user’s preferences. This ensures that predictions aren’t perceived as overbearing or moralizing. Anticipatory design must always allow users to refine or cancel predictions to fit their needs.
Feedback loops further enhance users’ agency. For example, a time-management tool might confirm a schedule based on the user’s productivity patterns: “Does this schedule work for you?” This allows users to accept, decline, or modify the suggestion, reinforcing control and ensuring that the system remains adaptable to their changing preferences.
User agency maintains user control and trust in AI systems. By providing transparency, allowing adjustments, and ensuring feedback loops, designers can create systems that guide users without overstepping while respecting autonomy and adaptability.
Personalization in Anticipatory Design
Personalization in anticipatory design ensures interventions align with the user’s unique context, behavior patterns, and stage of change, which avoids generic or intrusive interventions. Personalization refines prediction by factoring in the user’s recent behavior and context, such as extended inactivity during the day. Instead of broadly suggesting a morning workout, it might recommend targeted stretches to alleviate lower back discomfort caused by prolonged sitting. This approach makes interventions relevant and actionable, enhancing the likelihood of successful outcomes and long-term engagement.
Moreover, personalization helps in providing gradual learning and adjustments. For instance, a budgeting app might start by offering a general overview of spending and gradually increase the specificity of notifications or alerts as the user becomes more comfortable with the system. This gives users control over how much intervention they want, helping maintain their sense of agency and trust in the AI system.
Additionally, personalization avoids a one-size-fits-all experience. For example, a meal-planning app can personalize recipes based on dietary restrictions and goals, such as vegetarian or high-protein diets. By aligning meal suggestions with the user’s preferences, the app transforms from a generic tool into a trusted partner that adapts to the user’s unique needs, increasing engagement and effectiveness.
Consequently, personalization ensures that systems remain supportive of the user’s needs. Allowing users to customize nudges—such as adjusting the frequency and timing of reminders—ensures that nudges don’t become intrusive or moralizing, which helps prevent overwhelm and maintains user agency. Personalized interventions align with the user’s current goals and readiness for change, increasing the likelihood of behavior change.
Timely Interventions in Anticipatory Design
Nudging is a powerful tool that allows systems to guide users toward desired behaviors without being intrusive. By delivering timely and context-sensitive reminders or suggestions, designers can use nudges to significantly increase the likelihood of behavior change. For example, a smart thermostat might predict that lowering the temperature would save energy but doesn’t remind users about the financial benefits. A nudge—like a friendly prompt saying, “Lowering your thermostat by 2 degrees saves $15 this month!”—turns the prediction into a more meaningful action.
Similarly, a grocery app that anticipates that the user needs milk and adds it to the cart might get it wrong, especially if the user bought milk recently. A nudge like “Based on your usual patterns, you’ll run out in two days—shall we add it?” ensures that the prediction is contextually relevant. Users feel more engaged and in control of their decisions.
Another example is a calendar app that auto-schedules meetings for the user. Without a nudge, this might feel overbearing. However, “Would you like me to book a 15-minute buffer before your next meeting?” respects the user’s autonomy while still providing anticipatory assistance.
In the case of a budgeting app, nudges can simplify decision-making without overwhelming the user. For example, “Here’s where you could save $50 this month—tap to apply!” reduces cognitive load by offering a clear, actionable suggestion while avoiding excessive options or steps.
Nudges are most effective when they respect user autonomy, offering choices rather than mandates and ensuring that users are in control of their actions. By making predictions relevant and offering guidance in the right context, nudges connect prediction to action, enhancing user engagement and encouraging behavior change. When done effectively, nudging turns predictions into meaningful actions, making systems more intuitive, user-friendly, and empowering.
Navigating Challenges to Design for Cognitive Relief
The promise of AI-driven anticipatory and behavior-change design is its ability to transform technology from a source of stress to a partner that enhances human potential. By leveraging principles such as user agency, personalization, and timely interventions, designers can create systems that mitigate cognitive overload and empower users to make informed and meaningful choices. These principles, rooted in behavioral science, serve as a roadmap for reimagining user experiences in ways that prioritize human well-being.
However, realizing the potential of anticipatory design requires addressing significant challenges. Poor data quality, over-automation, and misalignment with user goals risk undermining trust and engagement. Data privacy concerns further complicate adoption as users demand greater transparency and control over their personal information. Additionally, the cost of designing, implementing, and maintaining these sophisticated systems can be prohibitive, especially for smaller organizations, posing a barrier to widespread adoption.
Designers, researchers, and organizations must adopt a holistic, human-centered AI approach by investing in data integrity, designing for transparency and explainability and ensuring that AI systems align with the complexities of human behavior to generate trust. By addressing these challenges, designers can unlock the transformative potential of anticipatory design to reduce cognitive strain, foster trust, and improve overall user experiences.
The future of human-centered AI must balance automation and autonomy with convenience and control. By making user experience the foundation of design, we can create technology that not only meets functional needs but supports mental and emotional well-being—a true testament to the power of thoughtful innovation.
Sources
Cerejo, Joana. 2024. “Why Anticipatory Design Isn’t Working for Businesses.” Smashing Magazine. https://www.smashingmagazine.com/2024/09/why-anticipatory-design-not-working-businesses/
Cerejo, Joana. 2025. “Designing the Future: Exploring the Dynamics of Anticipatory Design in AI-Driven User Experiences.” PhD diss. (unpublished), Faculty of Engineering of the University of Porto.
Fogg, B. J. 2009. “A Behavior Model for Persuasive Design.” In Proceedings of the 4th International Conference on Persuasive Technology, 1–7. New York. https://doi.org/10.1145/1541948.1541999
International Organization for Standardization. 2023. “Information technology—Artificial intelligence—Management system” (ISO/IEC standard no. 42001:2023). Retrieved from https://www.iso.org/standard/81230.html
Kore, Akshay. 2022. Applied UX Design for Artificial Intelligence Designing Human-Centric AI Experiences. Apress. https://link.springer.com/
Prochaska, James O., and Carlo C. DiClemente. 1983. “Stages and Processes of Self-Change of Smoking: Toward an Integrative Model of Change.” Journal of Consulting and Clinical Psychology 51, no. 3: 390–95. https://doi.org/10.1037/0022-006X.51.3.390
Schwartz, Barry. 2004. The Paradox of Choice, Why More Is Less. Harper Perennial. http://wp.vcu.edu/univ200choice/wp-content/uploads/sites/5337/2015/01/The-Paradox-of-Choice-Barry-Schwartz.pdf
Shapiro, Aaron. 2015. “The Next Big Thing in Design? Less Choice.” Fast Company. https://www.fastcompany.com/3045039/the-next-big-thing-in-design-fewer-choices
Thaler, Richard, and Cass Sunstein. 2008. Nudge: Improving Decisions about Health, Wealth, and Happiness. Yale University Press.
Joana Cerejo has been designing digital products since 2010. She is passionate about Artificial Intelligence, Data, and above all, Human Experience. Cerejo holds a PhD in Digital Media (UX for AI specialization) and was nominated for the 2021 Women in Artificial Intelligence Award for contributions to AI-driven user experiences.